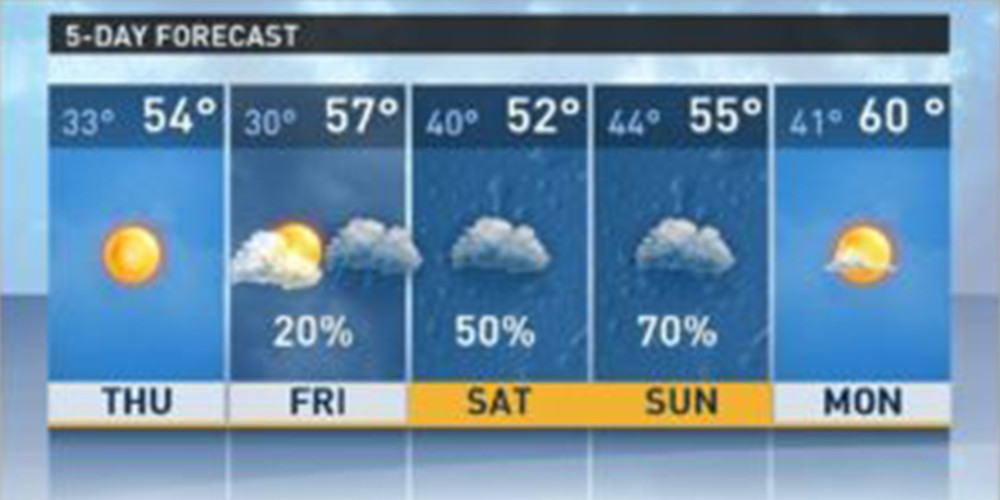
All crystal balls are somewhat broken
Forecasting is really, really hard. The more complex the system and the longer the forecast horizon, the harder it gets.
People complain about the accuracy of weather forecasts–a complex system–all the time. In reality, the accuracy of weather forecasts has improved dramatically in recent decades with the introduction of new measurement tools, models, and methods. The average error for a three-day forecast of high temperature dropped from 6 degrees in 1972 to about 3 degrees today. The average miss on a forecast of where a hurricane will hit from three days out declined from 350 miles in 1987 to about 100 miles today. One helps you decide to pack a sweater; the other could save your life.
While these improvements in accuracy are big advances, no forecast is perfect. The average error from a temperature forecast for 4pm today is pretty small. The error for tomorrow’s forecast is not that much larger. But the accuracy of the forecast starts to degrade beyond about three days and really tails off for the ubiquitous 10-day forecast. Acknowledging these limitations doesn’t negate the usefulness of an accurate forecast of the temperature over the next couple of days. You can always adjust what you wear and what you plan to do based on the more accurate shorter-term view of the weather.
Financial markets are another complex system that is notoriously difficult to forecast. An easy way to look like a fool is to try and predict the value of the S&P 500 one year from now. One study from the University of Waterloo and Boston College of 11,000 analysts in 41 countries revealed an average year-end market price forecast error of 30%. Not very helpful.
Still, there are areas within financial markets that are kinder to prognosticating, especially with the wider availability of data, computing power, and low transaction costs that are currently available. Publicly-traded Virtu Financial uses computing power, data, and models that attempt to estimate the price of thousands of financial instruments every second of the day as a market maker in equities, ETFs, futures, FX and other instruments around the world. It makes money not by making big bets as to where the British pound, Apple stock, or the price of soybeans will be six months or a year from now. It makes money by being only slightly more right than wrong over a very short forecast horizon , and repeats it thousands of times a day on relatively small amounts of capital. With low costs and high levels of automation, it can be a very profitable business model. A forecast is involved, but seeing the future in perfect focus is not required. The forecast is a tool in a process, not a magic signal.
Introducing truBeta™
Getting back to the weather analogy, assume you’re asked to estimate what the temperature will be at 4pm tomorrow. You have a choice of data to help you but can only pick one:
1. The temperature at 4pm today.
2. The temperature at 4pm two months ago.
3. The seasonal average temperature at 4pm.
Most people would choose option #1. Assuming that tomorrow will be about the same today–a “persistence forecast”–is not a bad guess. The temperature two months ago might be a completely different season. And while the seasonal average could be useful, the range of temperatures is still too wide to be an accurate representation of what is likely tomorrow.
Today’s temperature might be the best option in this scenario. But what if we had some alternatives? What if we could use all three? What if we could develop a process that uses multiple data sets and time horizons to produce an even better forecast? This past February we had a day over 70 degrees in New York City. It was a welcome respite from the bitter mid-winter cold but as a forecast for the next day’s weather, it was quite poor. By the time we woke up the next day it was back in the 30s, ending our small taste of summer. Putting that single day in context with patterns from the longer term can help filter some of the noise and drive a more accurate forecast.
Where are we going with this?
In one of our previous posts, we demonstrated some of the limitations of forecasting how stocks move with the market using a common statistical estimate called beta. Many investors are familiar with the concept and basic interpretation of beta. A stock with an estimated beta of 1.0 tends to vary in the same direction and magnitude as the market. A stock with a beta of 1.2 would be expected to vary 20% more than the market (higher volatility); one with a beta of 0.8 would tend to move 20% less than the market (lower volatility).
All that is required to calculate beta is a series of price returns for the stock and a market proxy such as the S&P 500. The beta coefficient is an output from a simple linear regression – the slope of the line created by regressing the returns of the individual stock on the returns of the market. Alternatively, beta can be calculated as the ratio of how the stock moves with the market (covariance) to the variance of the market.
Now let’s re-tool our question from above. Assume you’re asked to estimate a stock’s beta over the next quarter and can use only one of the following:
1. Intraday returns over the past couple of weeks.
2. Daily returns over the previous year.
3. Monthly returns over the past five years.
The optimal choice may not be so obvious. Option #3 happens to be the most common method of estimating beta, going back to a 1973 paper by Eugene Fama and James MacBeth. But it is difficult to see how the sensitivity of a stock to market moves 4 or 5 years ago has anything to do with how it might move over the next couple of months. A lot can change in five years in a market/industry/company that would affect how the stock behaves relative to the market.
Using more recent data and extrapolating a few months seems reasonable–it’s a version of the persistence forecast from above using today’s temperature to forecast tomorrow’s. But unlike the weather, financial markets have no set distance from the sun or rotation of the earth that drives much of larger trends in recent temperature. They are more fickle. Investors can collectively vote on where a stock will go that will cause more extreme gyrations in price that are not bound by spring, summer, winter, or fall.
Again, what if you could use all three? What if you could develop a process that uses the recent data yet grounds the estimate in some longer term patterns that might still offer some insight today?
At Salt Financial, we use rich data from the recent past to forecast how a portfolio is likely to behave in the near future, creating products designed to dynamically adapt to deliver consistent exposure to the underlying market for investors. This “micro-forecasting” technique lies at the heart of everything we do.
truBeta™ is the result of this micro-forecasting. It uses a blend of intraday, daily, and monthly historical return data to estimate beta over the next quarter, producing what we believe to be a more accurate estimate than traditional approaches.
We compared truBeta™ to some common methods that just use monthly, daily, or weekly returns to forecast beta. According to our study based on the top 1000 US stocks by market capitalization from 2004-2017, truBeta™ estimates were up to 52% more accurate than the traditional methods. Furthermore, truBeta™ showed more consistency in estimating very high and very low betas, which were areas of particular weakness for the traditional methods. Additional details on the methodology behind truBeta™ and its performance are available in our white paper located here.
Be the first to know when Salt publishes its latest analytics, research, and insights